The Future of Business: Leveraging Automatic Image Annotation for Growth
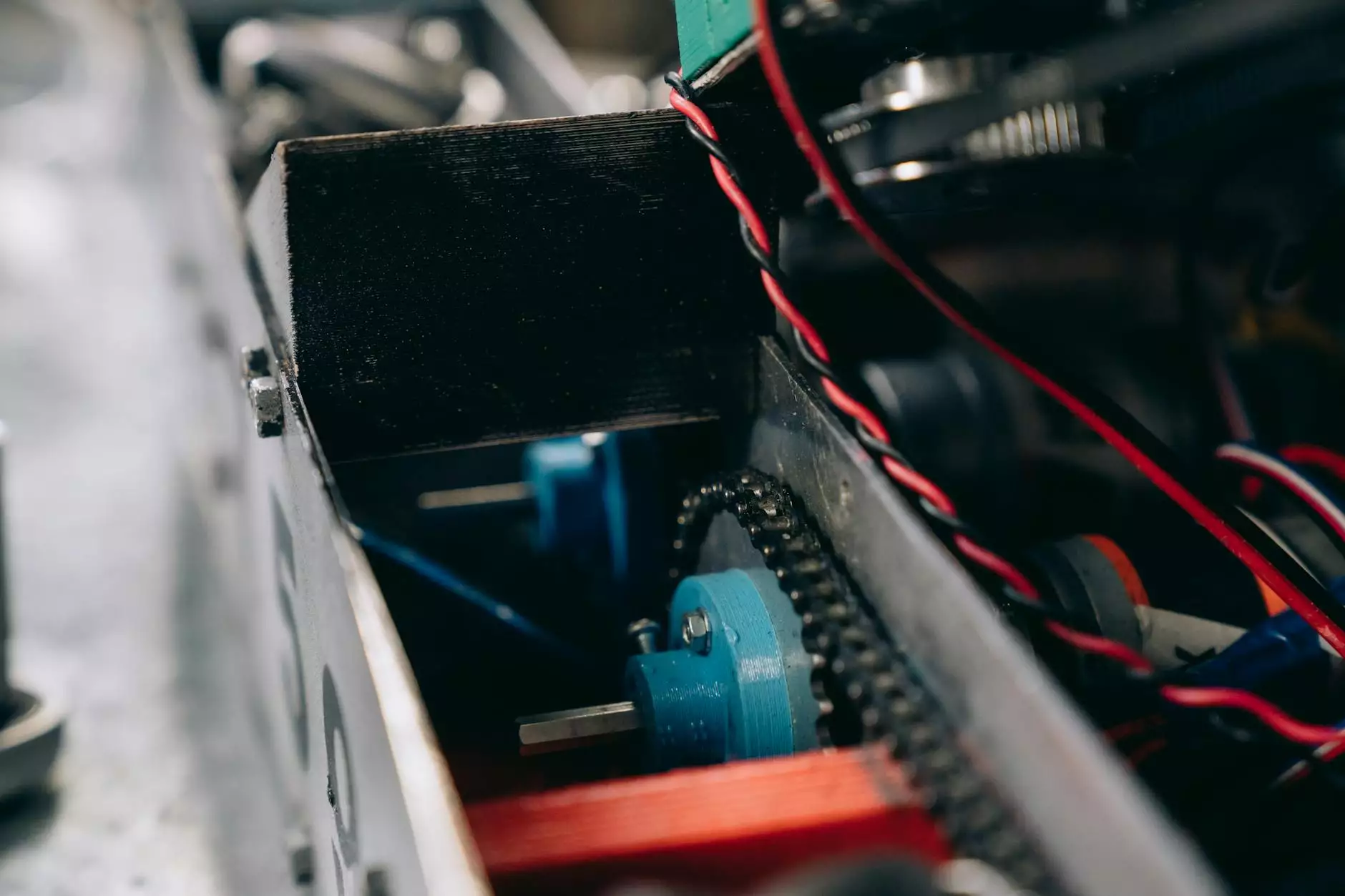
In the constantly evolving world of technology, businesses are continuously seeking innovative solutions to streamline operations, enhance productivity, and gain competitive advantages. One such groundbreaking technology that is paving the way for advancements in various industries is automatic image annotation. This article delves into what automatic image annotation is, its significance in the realm of software development, and how businesses can harness its power to thrive in today's digital landscape.
Understanding Automatic Image Annotation
Automatic image annotation refers to the process of automatically labeling images with descriptive tags or attributes using machine learning and artificial intelligence. Traditionally, image annotation required extensive manual effort, which was not only time-consuming but also prone to human error. However, with the advent of sophisticated algorithms and deep learning techniques, automatic image annotation has emerged as an efficient solution.
By utilizing vast datasets, machine learning models can learn to identify objects, scenes, and other characteristics within images. This automation not only improves accuracy but also enables businesses to annotate large volumes of images quickly and efficiently.
The Importance of Automatic Image Annotation in Today’s Business Environment
As businesses increasingly adopt visual data in their operations, the demand for effective image annotation has never been more crucial. Here are several reasons why automatic image annotation is vital for modern enterprises:
- Enhanced Productivity: By automating the image annotation process, companies can save significant time and resources that would otherwise be spent on manual labeling tasks.
- Increased Accuracy: Advanced algorithms reduce the likelihood of errors that often occur in manual processes, leading to more reliable data and insights.
- Scalability: Businesses can scale their operations significantly by handling a larger volume of images without a proportional increase in labor costs.
- Better Insights: Accurate image annotations can enable businesses to derive more profound insights from visual data, allowing for data-driven decision-making.
Applications of Automatic Image Annotation in Software Development
The applications of automatic image annotation in software development are vast, affecting various segments of the industry. Here are some key applications:
1. Computer Vision and Image Recognition
Automatic image annotation plays a crucial role in the field of computer vision. Companies developing image recognition software rely on high-quality annotated datasets to train their models. This training allows systems to accurately identify and classify images, which is essential for applications such as facial recognition, object detection, and more.
2. Autonomous Vehicles
The automotive industry has seen tremendous advancements with the integration of automatic image annotation. Autonomous vehicles use annotated images to recognize obstacles, traffic signs, and lane boundaries, ensuring safe navigation on the roads.
3. E-Commerce and Retail
For online retailers, automatic image annotation enhances product categorization and searchability. By accurately annotating product images, consumers can enjoy a more intuitive shopping experience, ultimately leading to higher conversion rates.
4. Medical Imaging
The healthcare sector benefits significantly from automatic image annotation. In radiology, annotated images of scans can help physicians in diagnosing diseases more accurately and swiftly, improving patient care.
The Process of Automatic Image Annotation
Understanding how automatic image annotation works is essential for businesses looking to implement this technology. Here’s a step-by-step overview:
Step 1: Data Collection
The first step involves collecting a diverse set of images pertinent to the specific business needs. The quality and quantity of the dataset directly impact the annotation results.
Step 2: Preprocessing
Images may require preprocessing, such as resizing or normalization, to ensure they meet the input requirements of the machine learning model.
Step 3: Model Training
A machine learning model is trained on the annotated dataset. This process involves adjusting the model parameters so it can learn to predict labels based on image features.
Step 4: Annotation
Once trained, the model can automatically annotate new images. The accuracy of the annotations can be continuously improved by refining the model through feedback and additional training data.
Challenges in Automatic Image Annotation
While automatic image annotation presents numerous benefits, there are also challenges that businesses must be aware of:
- Data Quality: The accuracy of automatic annotation is heavily reliant on the quality of the training data. Poor-quality data can lead to incorrect annotations.
- Model Bias: If the training dataset is biased or not representative, the model may produce skewed results, limiting its effectiveness.
- Integration: Integrating automatic image annotation into existing workflows can be complex, requiring technical expertise and resources.
- Cost: Depending on the technology stack, initial implementation costs can be high, although these can be offset by long-term savings.
Future Trends in Automatic Image Annotation
The field of automatic image annotation is rapidly evolving, and several trends are likely to shape its future:
1. Advanced Deep Learning Techniques
As deep learning continues to advance, we can expect automatic image annotation to become even more sophisticated. New algorithms will enable more nuanced understanding and labeling of complex images.
2. Integration with Other AI Technologies
Automatic image annotation is increasingly being integrated with other AI technologies, such as natural language processing, to create richer datasets that can also include metadata, enhancing machine learning applications.
3. Democratization of Technology
With the rise of accessible machine learning frameworks, businesses of all sizes can leverage automatic image annotation. This democratization will empower more companies to innovate and utilize image data effectively.
How KeyMakr Can Support Your Automatic Image Annotation Needs
At KeyMakr, we specialize in software development solutions that harness the power of automatic image annotation. Our expertise lies in developing robust, scalable systems that cater to the unique needs of businesses across various industries. Here’s how we can help:
- Custom Solutions: We provide tailored software solutions that fit your specific annotation needs and business objectives.
- Expert Consultation: Our team of experienced professionals will guide you through the implementation process, ensuring a seamless integration.
- Continuous Support: We offer ongoing support and maintenance to adapt your automatic image annotation system as your business grows and evolves.
Conclusion
In an era where visual data is becoming increasingly prominent, businesses cannot afford to overlook the potential of automatic image annotation. By embracing this technology, companies can enhance efficiency, improve accuracy, and unlock valuable insights that drive growth. As the future unfolds, those who invest in automatic image annotation will be well-positioned to lead in their respective fields. KeyMakr is here to assist you every step of the way, providing innovative software development solutions tailored to your needs.