Harnessing the Power of Data Labeling Tools to Transform Business in Software Development
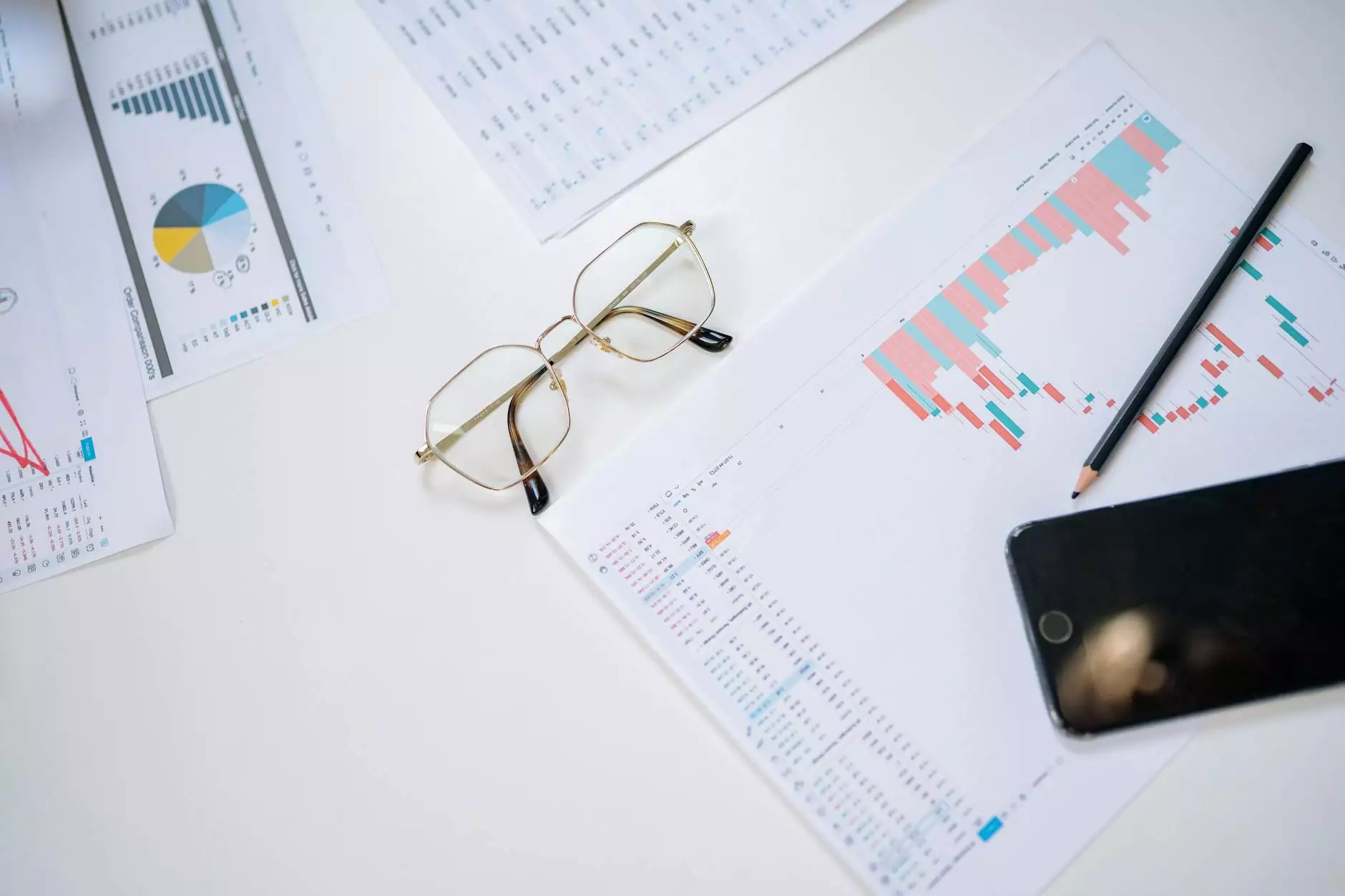
In an era where artificial intelligence (AI) and machine learning (ML) are redefining industries, the foundation of successful AI models lies in high-quality data. At the heart of this process is the data labeling tool, an essential component that enables businesses to prepare and annotate vast amounts of unstructured data accurately. For companies engaged in software development, leveraging the right data labeling tool can dramatically enhance model accuracy, reduce development time, and gain a competitive edge in the marketplace.
Understanding the Significance of Data Labeling in Modern Software Development
Data labeling is the systematic process of annotating data—be it images, videos, text, or audio—to enable machine learning algorithms to interpret and learn from it effectively. Without precise labeling, models would lack context and reliability, leading to subpar performance and unreliable predictions.
In software development, particularly in AI-driven projects, data labeling tools serve as the backbone for creating training datasets that are both accurate and comprehensive. High-quality labeled data ensures that AI models can identify patterns, recognize objects, understand language nuances, and make decisions with a high degree of certainty.
Key Features of Advanced Data Labeling Tools for Business Success
When selecting a data labeling tool, businesses should prioritize features that enhance efficiency, accuracy, and scalability. Some of the most critical features include:
- Intuitive User Interface: Simplifies the annotation process, reducing training time and minimizing errors.
- Support for Multiple Data Types: Images, videos, text, and audio to accommodate diverse project requirements.
- Automation Capabilities: AI-powered automation for repetitive tasks accelerates workflow and maintains consistency.
- Collaboration Features: Enables teams to work seamlessly, track progress, and ensure quality control.
- Integration with ML Pipelines: Seamless connectivity with existing software development workflows and frameworks.
- Quality Assurance Tools: Validation mechanisms to ensure that labeled data meets strict standards.
- Security and Data Privacy: Protect sensitive data, especially in regulated industries like healthcare and finance.
The Business Advantages of Implementing a High-Quality Data Labeling Tool
Adopting the right data labeling tool can unlock numerous benefits for businesses engaged in software development. These advantages include:
1. Accelerated Development Cycle
Automated and streamlined annotation processes enable teams to prepare datasets more quickly, reducing project timelines and accelerating product deployment.
2. Enhanced Model Accuracy
Precise and consistent data labeling leads to more robust AI models, which in turn improves performance, reliability, and user experience.
3. Cost Efficiency
Efficient tools reduce manual effort and lower labor costs, allowing organizations to allocate resources more effectively across projects.
4. Competitive Differentiation
Having access to high-quality labeled data positions a business as an innovator, enabling it to develop superior AI solutions that stand out in the market.
5. Scalability for Growing Data Needs
Modern data labeling tools are built to scale effortlessly, handling increasing data volumes without compromising quality or speed.
Integrating Data Labeling Tools into Your Software Development Workflow
To maximize the benefits, organizations should integrate data labeling tools seamlessly into their existing workflows. This involves several best practices:
- Assessment of Requirements: Understand the specific data types and labels needed for your projects.
- Choosing the Right Tool: Select a data labeling tool that aligns with your technical stack and scalability plans.
- Training and Onboarding: Ensure that your data annotators are well-trained to maintain consistency and quality.
- Quality Control Processes: Implement review cycles and validation checks to uphold high annotation standards.
- Iterative Improvements: Use feedback to refine annotation guidelines and improve data quality over time.
Innovative Trends in Data Labeling Technology
The field of data labeling is constantly evolving, driven by technological advancements. Recognizing these trends helps businesses stay ahead:
1. AI-Assisted Labeling
Leverages machine learning algorithms to pre-annotate data, significantly reducing manual effort and increasing consistency.
2. Crowdsourcing Platforms
Tap into global talent pools for large-scale annotation tasks, while maintaining quality through validation workflows.
3. Automated Quality Assurance
Employs AI to automatically detect errors and discrepancies, ensuring data integrity without extensive manual review.
4. Customizable Labeling Interfaces
Allows for tailored annotation workflows that match specific project needs, improving speed and accuracy.
Key Considerations for Selecting a Data Labeling Tool for Your Business
Choosing the optimal data labeling tool requires thorough evaluation of several factors:
- Ease of Use: User-friendly interfaces reduce training time and errors.
- Compatibility: Ensure integration capabilities with your existing development and AI frameworks.
- Support and Maintenance: Reliable customer support and regular updates to adapt to evolving needs.
- Pricing Model: Transparent costs aligned with your project budget and scale.
- Security: Robust data protection measures for sensitive or proprietary information.
- Community and Ecosystem: Access to user communities and third-party extensions enhances capabilities.
Success Stories: How Leading Tech Companies Thrive Using Data Labeling Tools
Many industry leaders have harnessed state-of-the-art data labeling tools to transform their AI initiatives. For example:
- Autonomous Vehicles: Companies like Tesla and Waymo utilize sophisticated labeling platforms to annotate millions of images and sensor data for training self-driving systems.
- Healthcare AI: Accurate labeling of medical images and clinical notes improves diagnostic accuracy and treatment planning.
- Retail and E-commerce: Enhanced image and text annotation enables better product recommendations and personalized shopping experiences.
Conclusion: The Future of Business in Software Development with Data Labeling Technology
As AI continues to evolve and permeate various business sectors, the importance of high-quality data labeling tools becomes increasingly evident. They are not merely auxiliary components but strategic assets that empower organizations to develop smarter, faster, and more reliable software solutions.
Businesses that prioritize investing in advanced data annotation solutions will unlock unprecedented opportunities for innovation, growth, and market leadership in the digital age.
For all your data labeling tool needs in the domain of software development, explore the comprehensive solutions offered by keymakr.com, designed to elevate your AI projects to new heights.